Data processing pipeline integration with LLMs benefits
Large Language Models (LLMs) have been getting a lot of attention (for good reason!) lately, but not many have seen practical implementations beyond chat bots. A key reason for this trend is the lack of data availability for the LLMs. Since chat bots only require user input (and, potentially, a vector database), minimal data engineering is required. This means chatbots can be quick to implement but may ignore a company’s most valuable resource – data.
7Rivers believes combining Snowflake container services with LLMs will accelerate the integration of LLMs in data processing pipelines and make data more readily accessible for the LLMs. By integrating data processing pipelines with LLMs we can:
- Simplify data architectures
- Enable data-first event driven architectures
- Eliminate data egress
- Improve collaboration between DE and ML Engs through sharing a common platform (Snowflake)
- Centralize MLOps and data sources
A simple architecture can get you started on integrating LLMs and your data processing pipelines. This architecture takes advantage of Snowflake container services to run everything natively on Snowflake. This eliminates any data egress and helps centralize workflows on a single platform. In the architecture diagram below, additional Snowflake native features are being used as well.
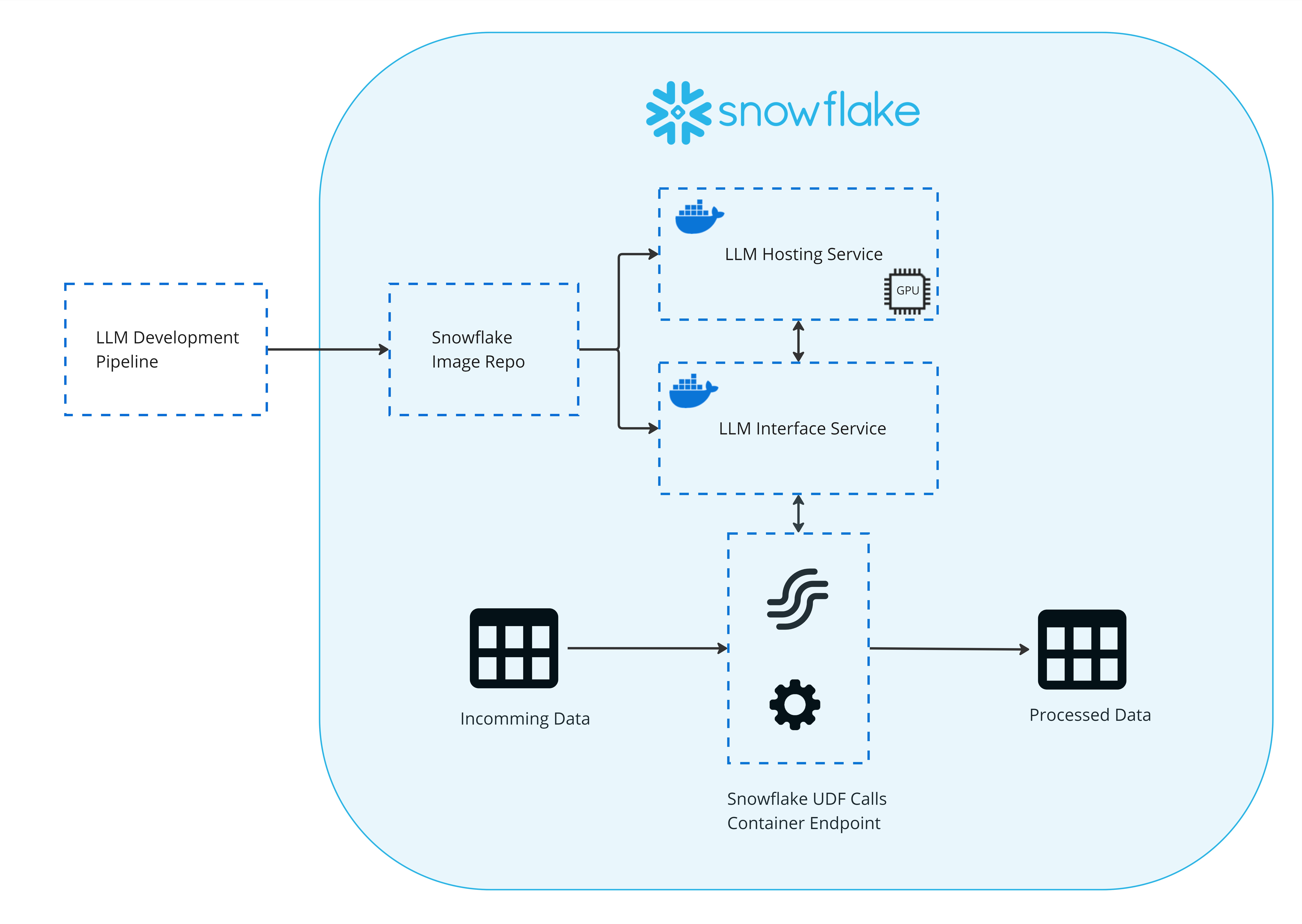
These Snowflake native features include container services, image registry, UDFs, streams, and tasks. The LLM development process is abstracted away, and the focus is on the implementation of the LLM natively in a Snowflake pipeline. Using streams and tasks for incremental data processing is a common Snowflake design pattern, and using a UDF to call the LLM interface fits naturally in this pattern. A separate LLM interface from the LLM hosting service to allow for greater flexibility, including implementing additional functionalities without wasting GPU resources. Furthermore,additional vector database services could be hosted alongside the LLM hosting service which the interface service could access to perform RAG if required.
Example
In this case, product reviews are examined as they are streamed into Snowflake and loaded continuously using Snowpipe. The reviews need to be classified based on a positive or negative sentiment regarding a specific metric such as product quality, along with the reason behind the classification. The classified product reviews will then be saved to a table where they can later be reviewed.
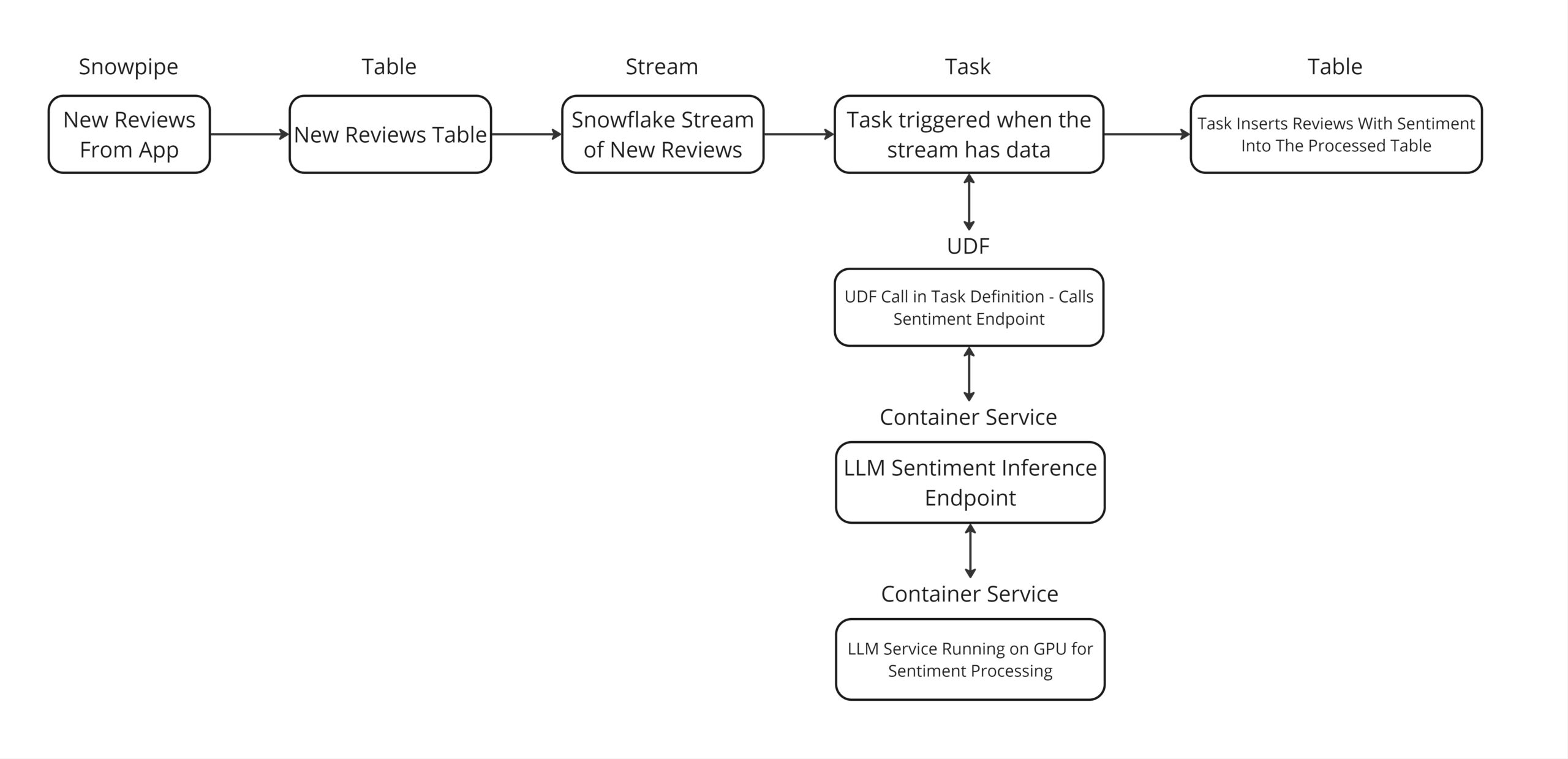
A benefit of this pipeline is that there is no overhead pulling the data out of Snowflake into another service to do the inference. The pipeline is contained in Snowflake and executed in an event-driven way. This enables Data Scientists and ML Engineers to more easily deploy their pipelines in production because they don’t need to worry about setting up any infrastructure outside of Snowflake. Spinning up infrastructure outside of Snowflake would typically require the aid of a DevOps team member and slow delivery velocity.
Furthermore, since the endpoint is exposed as a SQL UDF, Data Engineers will have no trouble using the UDFs the ML Engineers expose into their own pipelines. It is common for machine learning teams to pull data out of Snowflake into a separate pipeline that runs on a third-party service, which can cause issues with data like the pipeline being out of sync with the Snowflake source of truth. By keeping everything in Snowflake, not only are there more opportunities to collaborate with the data engineering team, but it ensures the data machine learning teams’ reference is the source of truth.
Conclusion
Snowflake container services make it easy to use LLMs as part of your data pipelines, allowing you to unlock new insights from your data without worrying about complex architectures. If you’d like to learn more about implementing this architecture or how we deployed this pipeline reach us at https://7riversinc.com/contact-us/