One of the biggest challenges in a rapidly growing enterprise is the disconnect between the IT-driven data infrastructure and business units, and their processes. When data operations are decoupled from a cross-domain semantic information framework, we often see a progression of symptoms which result in pain points for the business and missed potential for deriving the full value of their data.
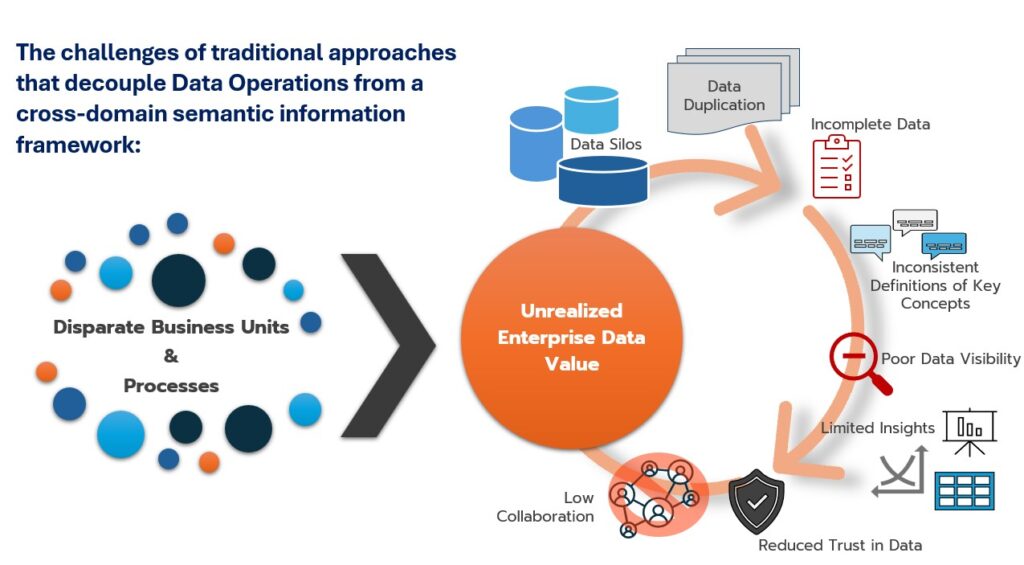
Traditional data warehousing approaches have primarily focused on storage, governance, and managing data volume. However, augmenting these capabilities with a domain-driven architecture is key to building a modern, data native enterprise. Investing in this foundational strategy ensures that data is closely aligned with business operations and decision-making processes. Not sure where to start? Here’s an incremental approach:
Phase 1: Domain-Driven Information Architecture
Enterprise Data Assessment:
- The journey begins with a comprehensive domain data assessment. This involves mapping interrelated data to business events and processes while establishing governance policies grounded in a thorough understanding of these processes. Data entities are defined based on their semantic relationships to key business operations.
Cross-Functional Business Alignment & Defining a “Golden Record”:
- Establishing an operations-focused information infrastructure and knowledge repository is essential for creating a cohesive data environment. This includes building a business data catalog and glossary, developing shared taxonomies, and ensuring consistent definitions of critical business concepts and attribute requirements across all business units. Such alignment promotes collaboration and reduces inconsistencies across domains.
- A shared understanding of data entities enables the creation of “golden records” that serve as the single source of truth for critical business data. These records, built on consistent definitions and shared taxonomies, ensure data integrity and reliability across the organization.
Enhanced Data Governance:
- Providing a 360-degree view of business processes significantly enhances data governance efforts. This holistic perspective allows for better oversight, improved data quality, and increased accountability throughout the organization.
- By identifying and collaborating with the business units and stakeholders who serve as stewards for each core domain, data infrastructure teams can ensure consistency and reliability in the treatment of data at every step in the pipeline from ingestion to output.
Phase 2: Data Foundations
With a solid understanding of business domains and concepts in place, organizations can turn their focus to building a robust data and information infrastructure. This phase prioritizes creating a reliable domain-driven foundation for managing and utilizing data effectively:
- Optimizing Data Pipelines: Streamlined and efficient data pipelines are essential for ensuring that data flows smoothly from source systems to end users. This optimization minimizes bottlenecks, reduces latency, and supports real-time decision-making.
- Establishing a Shared Repository: A centralized repository for all raw domain data creates a single access point for data storage and retrieval. This shared repository ensures consistency, reduces duplication, and enhances the overall accessibility of data across the organization.
- Master Data Management (MDM): Implementing MDM practices provides visibility into data lineage, traceability, and quality. By understanding where data comes from and how it is processed, organizations can ensure the accuracy and reliability of their datasets. This becomes especially important when adopting AI and ML capabilities.
- Harmonizing Business Logic: Standardizing and harmonizing business logic across the enterprise establishes a single source of truth for each data entity. This ensures consistent usage of data definitions and calculations, eliminating discrepancies and improving trust in data.
- User-Facing Information Marts: Creating information marts that interrelate cross-domain data elements gives users access to meaningful insights. These marts act as curated datasets tailored to specific business needs, bridging the gap between raw data and actionable intelligence.
- Parallel Data Documentation and Cataloging: Comprehensive documentation and cataloging of data assets occur alongside infrastructure development. This parallel effort ensures that data is well-documented, discoverable, and understandable, laying the groundwork for advanced analytics and AI initiatives.
Phase 3: Connecting business domains to augmented analytics and AI capabilities
Business Information Marts for Cross-Domain Insights:
- Information marts serve as a central repository for interrelated insights drawn from multiple business domains. These marts provide stakeholders with a comprehensive view of organizational performance, enabling more informed strategic planning and operational adjustments.
- Additionally, they support AI-infused applications and intelligent experiences by aggregating insights that empower cross-functional teams with actionable intelligence. As a foundation for Enterprise LLMs and Data Native™ applications, information marts facilitate the development of advanced AI-powered solutions and promote organization-wide collaboration. By driving data collaborations, these marts enable real-time sharing of insights across departments, accelerating decision-making and fostering better alignment with strategic objectives.
With a scalable data infrastructure in place, organizations can now unlock the full potential of their data ecosystem with advanced analytics and artificial intelligence (AI) capabilities:
Optimized Collaboration through Standardized Analytics:
- Organizations will reap the benefits of initial mapping and alignment efforts in the initial phase. The uniform interpretation of key metrics and concepts across the enterprise, and a streamlined, scalable data infrastructure result in enhanced data interoperability and seamless data exchange across business units
- Advanced analytics platforms act as connective tissue, linking various business processes and operations. By “connecting the dots” and using a shared language and single source of truth for key data data domains and KPI’s, these platforms can provide a holistic view of the enterprise, enabling proactive problem-solving and continuous improvement.
- The structured semantic frameworks implemented during the initial phases help to contextualize data within a coherent ecosystem. These frameworks enhance the value of data by supporting robust AI modeling and process automation, ultimately driving operational efficiency and innovation.
Governance in the Age of GenAI:
- Aligning business definitions through augmented glossaries and knowledge platforms ensures that all teams continue to operate with a unified understanding of core concepts. This alignment must be an ongoing effort to maintain reliability of analytics outputs.
- As generative AI (GenAI) becomes an integral part of business operations, organizations must establish guardrails to balance innovation with governance. This involves defining clear policies and practices for managing model metadata, tracking lineage, and implementing monitoring systems to ensure compliance and ethical use of AI.
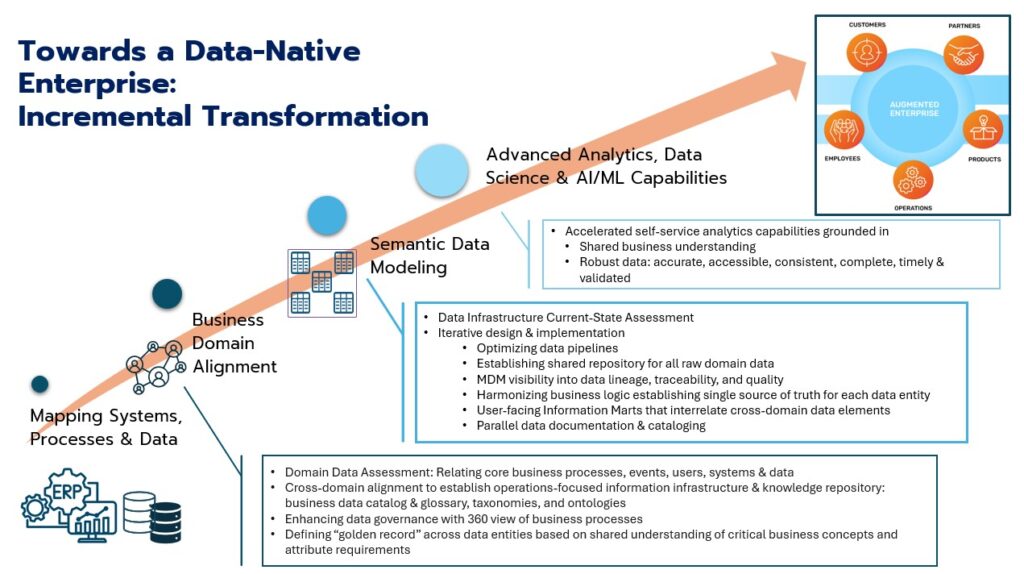
Achieving AI readiness and advancing data maturity can be a complex journey, but you don’t have to navigate it alone. At 7Rivers, we specialize in empowering businesses in their journey to building a data native enterprise. Whether you’re starting with domain-driven architecture, or building advanced AI capabilities, we can help you design a roadmap to success. Reach out to 7Rivers today to take the first step!